
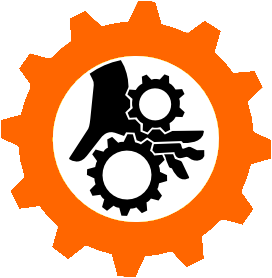
They probably got fed up with a broken system giving up it’s last shreds of legitimacy in favor of LLM garbage and are trying to fight back? Getting through an editor and appeasing reviewers already often requires some compromises in quality and integrity, this probably just seemed like one more.
Ah, you see, you fail to grasp the shitlib logic that the US bombing other countries doesn’t count as illegitimate violence as long as the US has some pretext and maintains some decorum about it.